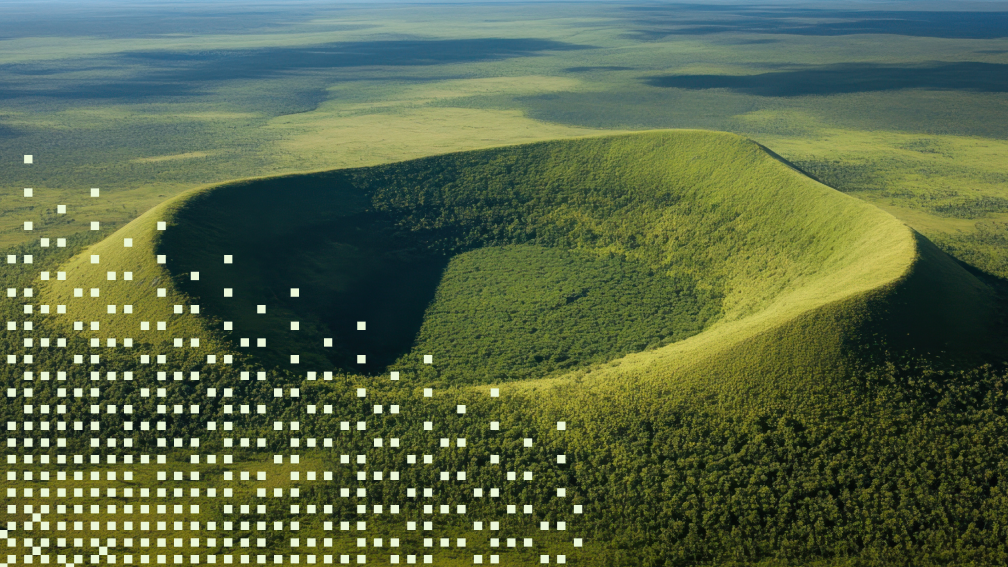
May 14, 2025 • 11 min read
Navigate AI governance and regulatory compliance in finance
The AI and financial landscape is undergoing a seismic shift. As artificial intelligence becomes the backbone of financial innovation, we need robust AI governance to ensure technology serves innovation, safety, and regulatory compliance. At the intersection of emerging tech and rigorous oversight, financial institutions must be at the forefront of rewriting the rules of engagement, particularly AI engagement.
AI governance and strategy
I hear business leaders demand, “Put AI in business operations.” They don’t ask why they need it, what they need it for, or how they will leverage it. This strategy is short-sighted at best. Any great business starts with AI strategy and governance before putting AI into its business operations. An AI Governance model aims to cover the following elements:
- Executive Accountability: It’s key to establish C-Suite ownership of core AI initiatives, particularly those that are enterprise-wide, defining clear escalation paths for key decisions and AI concerns, as well as providing space for board-level oversight, when needed.
- Core AI Pillars: An effective governance framework must provide and cover key core pillars that the business will use for AI, such as accountability, third-party oversight, data governance and training, responsible AI, and regulatory compliance.
- Core Business Cases for AI: Defining and starting with a tangible strategic direction and some specific use cases for leveraging AI for the business is fundamental for success. Here’s an AI template that covers examples of AI strategy along with real-world AI use cases in cyber.
- Data Governance and Integration: This part of the governance framework provides a guideline and establishes some key ground rules on how data should be used in all AI training sets while documenting source, permissions, quality metrics, and potential biases.
- Regulatory Compliance and Responsible AI: Establishing core AI principles for responsible innovation and ethical, transparent, and unbiased use of AI while ensuring compliance with various regulatory requirements is key.
AI use cases in finance and why AI governance matters
As per the OECD’s 2024 report on “Regulatory approaches to Artificial Intelligence in finance,” the top 3 use cases of AI in finance are:
- Effectiveness of customer service
- Fraud detection and/or market abuses
- Credit and insurance underwriting
The use of AI requires a strong governance model, particularly in critical sectors like finance. What this means in practice is that the key elements of responsible AI are taken into account in every AI-related decision, especially those that are risky decisions. You want key responsible practices such as safety, security, transparency, fairness, and accountability, asked and answered.
Use cases of AI, particularly in the finance sector require a strong governance model where Responsible AI is taken into account in every AI-related decision and is based on the key characteristics of safety, security, transparency, fairness, and accountability.
Understanding the new regulatory paradigm
Europe is leading the global charge in AI regulation. Nevertheless, we are still behind in regulating AI to a point where it provides a robust, practical, and efficient framework whilst innovation thrives.
We need regulated AI that supports innovation in a way that is:
- Efficient. Technological integration and advancement to streamline processes at scale and reduced costs
- Responsive. Leaner, faster and robust business model with financial products that are hyper-tailored to improve customer experience
- Accessible. Novel financial instruments not only serving the broader market but the underserved market segments
Three key regulations, among many others, are redefining how financial institutions need to approach AI.
DORA: Digital operational resilience act
The Digital Operational Resilience Act is about the resilience of the financial economy, and mandates the implementation of controls to strengthen the financial sector's digital defenses through 5 key pillars:
- Information sharing
- ICT risk management
- Robust incident reporting
- Stringent third-party risk controls
- Regular threat-led penetration testing
AI is a double-edged sword. Despite changing the finance sector beyond imagination, AI introduces risks. Through its five key pillars and a strong focus on resilience, directives like DORA are a great foundation for secure, stable, and resilient financial AI systems amidst unpredictable and growing challenges with emerging tech.
The EU AI Act: A global AI regulation
The EU AI Act is one of the world’s first comprehensive AI laws. It introduces a risk-based approach to AI governance. For financial institutions, this means stricter classification of AI systems based on potential risks, mandatory transparency and explainability, prohibition of certain unacceptable AI practices, stronger regulations in case of high-risk systems, and human oversight. The law categorizes AI applications by risk level, for example as follows:
- Unacceptable Risk AI: Banned outright (e.g., AI for social scoring)
- High-Risk AI: Subject to strict regulation (e.g., AI for credit scoring, fraud detection, and risk assessment)
- Limited & Minimal Risk AI: Lower compliance requirements (e.g., AI-powered chatbots).
GDPR & AI: The battle over data privacy
AI thrives on data. As they say “Garbage In, Garbage Out” but GDPR (General Data Protection Regulation) places strict limits on how data can be processed, stored, and used. Here are some real-world examples that map regulations on AI from a GDPR perspective:
- AI-driven profiling and automated decisions must be explainable. Customers have the right to know why they were denied a loan.
- Explicit consent for AI-driven decisions. Banks must ensure customers understand how AI is being used and consent to have their data used by AI or trained for AI.
- The “Right to be Forgotten” also applies to AI training data. AI models must be retrainable or deletable if required. It’s not easy, but it needs to be possible and doable under GDPR.
For AI developers in finance, GDPR forces a balance between innovation and individual rights.
Practical implications for financial institutions
With DORA, the EU AI Act, etc., the regulatory landscape today demands more than mere compliance, and rightly so. While AI is powerful, any risky AI decision without oversight, accountability, and safety can wreak havoc. Leveraging AI requires fundamentally reimagining the underlying strategy, governance framework, and implementation of AI in a safe, secure, robust, unbiased, and ethical way. Financial institutions must now:
- Develop transparent AI systems. Most AI are black-boxes. But that needs to change. Regulations like DORA and The EU AI Act demand a level of transparency. With the need for open-source models and explainability rising, regulators now demand AI systems that can explain their decision-making processes, especially in high-risk use cases like credit scoring. What factors was the decision based on? How did it come to that conclusion? What other alternative decision paths did the AI model consider?
- Implement robust governance frameworks. This means creating cross-functional teams like cyber, legal, and fraud teams working together in a fusion format that blends technical expertise with legal, anti-fraud, and ethical oversight, supported by a strong and tangible AI strategy. The goal is to create AI systems that are not just powerful but fundamentally trustworthy. As per KPMG’s survey, three in five people are wary about trusting AI systems.
- Do continuous monitoring and risk assessment. Your audit teams need to take into consideration not only financial controls, but also anti-fraud controls, AI controls related to AI risks, and security controls, followed by regular and frequent checks to manage any potential bias, unfair use, and legal ramifications, as outlined in the UK’s National AI strategy.
AI governance starts with a holistic understanding of the use cases and AI risks, and asking the right questions about AI. Building AI-based business operations without strategy or governance in place is like driving a Ferrari blindfolded with an autopilot but no safety mechanisms or insurance in place. You never even consider driving like that. Driving innovation with technologies like artificial intelligence requires a similar approach ,i.e. well-defined strategy, a strong governance model, and continuous monitoring and auditing for regulatory compliance.
You may also like to read
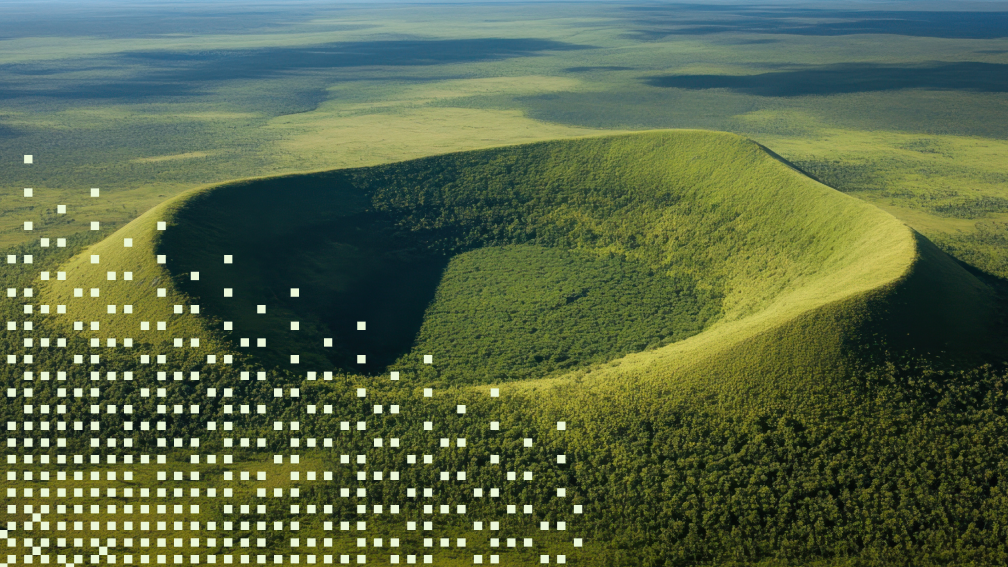
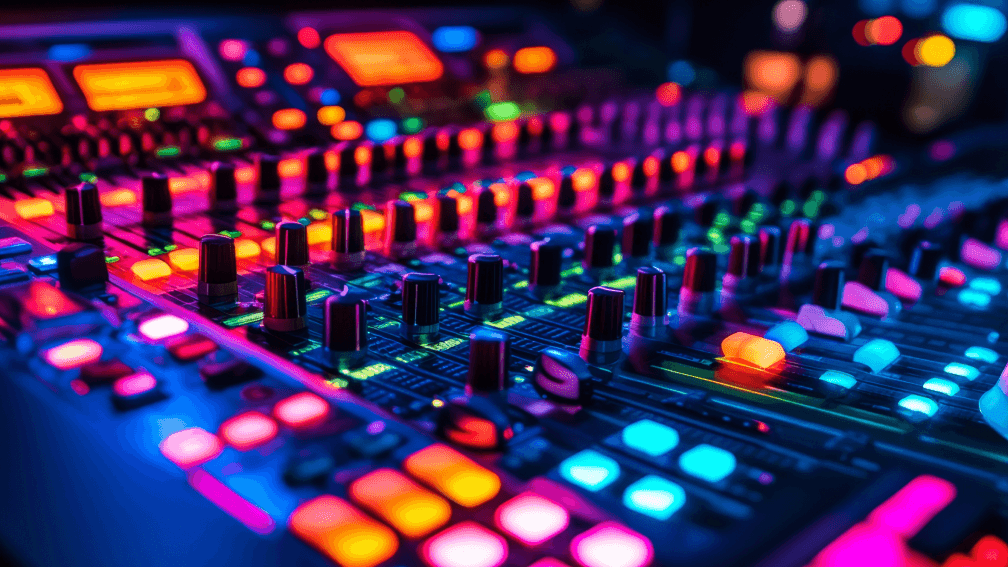
Commanding Compliance: Demystify the Common Control Set
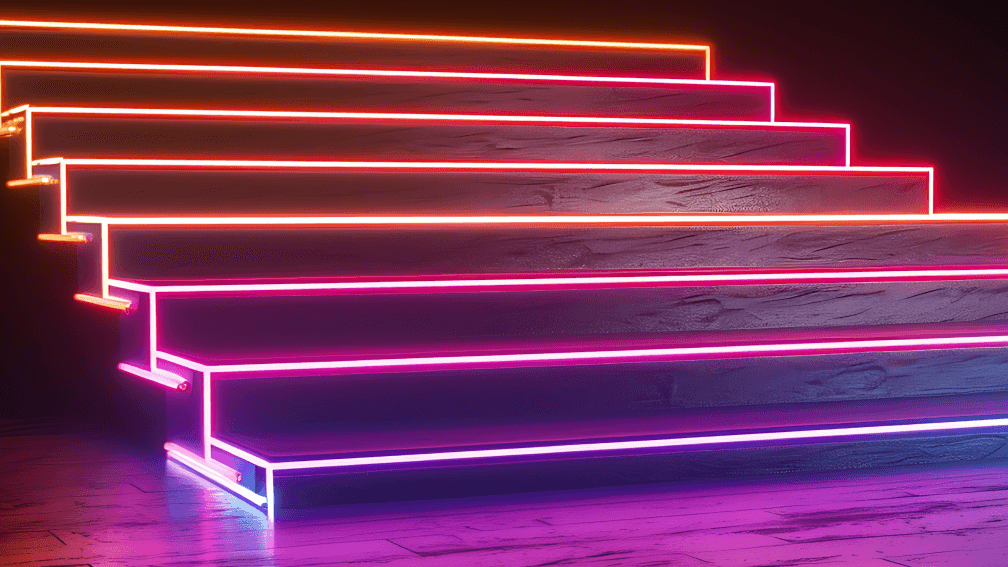
Practical Steps for Applying NIST CSF 2.0 to Third-Party Risk Management
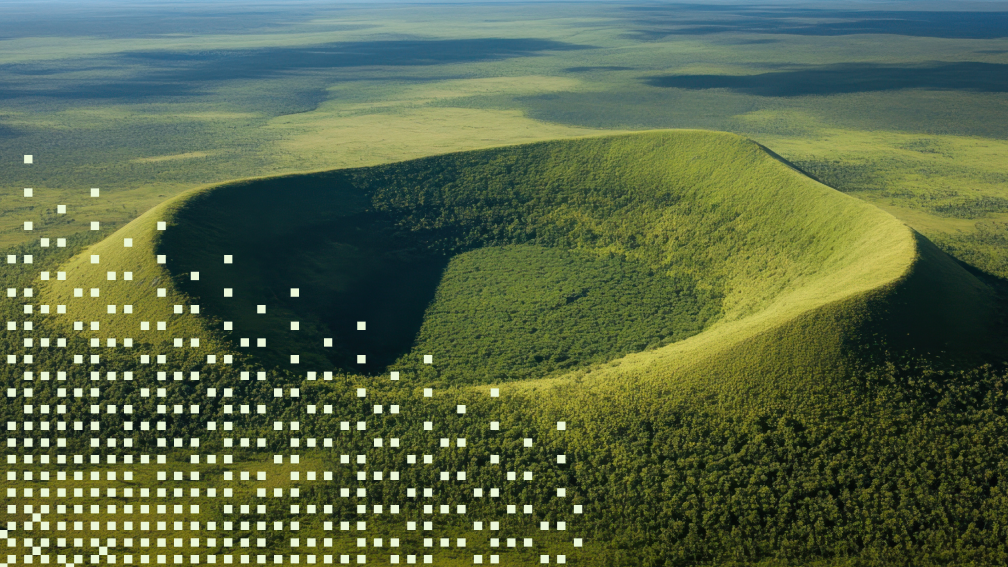
AI Governance: Automated Control Testing for ITRC
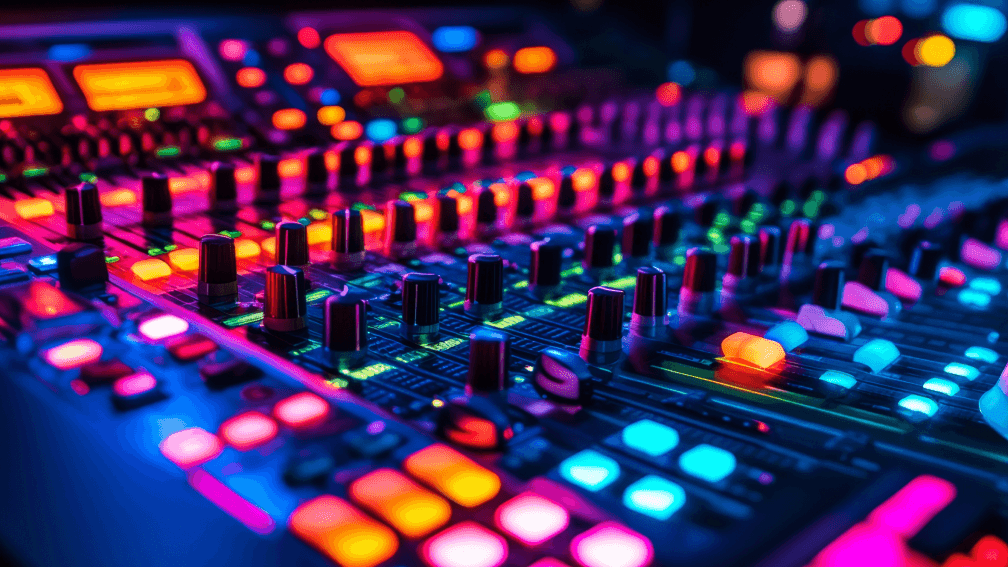
Commanding Compliance: Demystify the Common Control Set
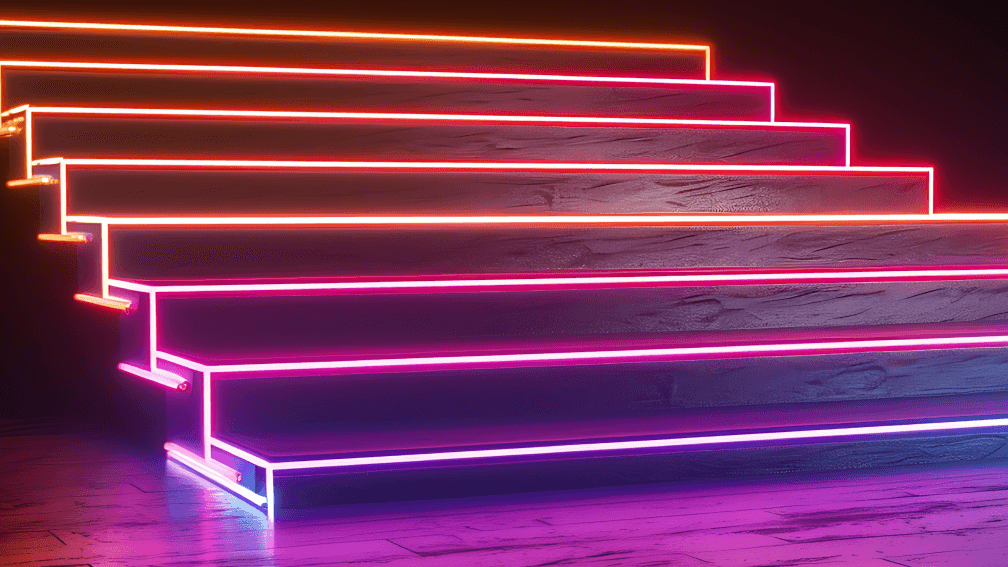
Practical Steps for Applying NIST CSF 2.0 to Third-Party Risk Management
Discover why industry leaders choose AuditBoard
SCHEDULE A DEMO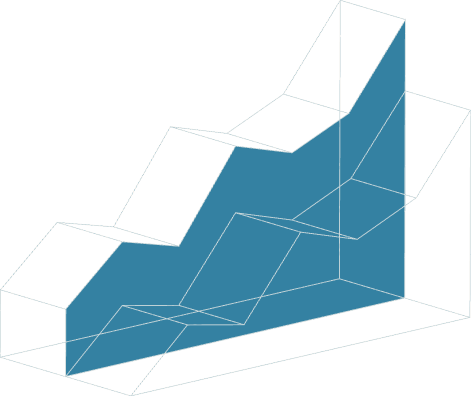
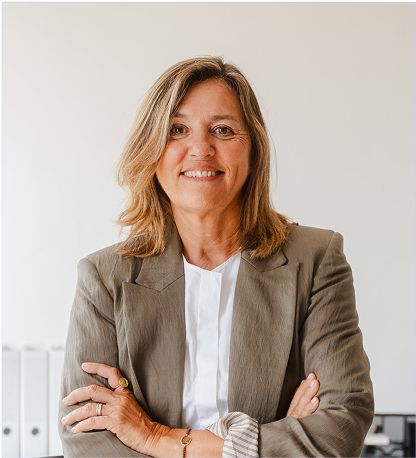